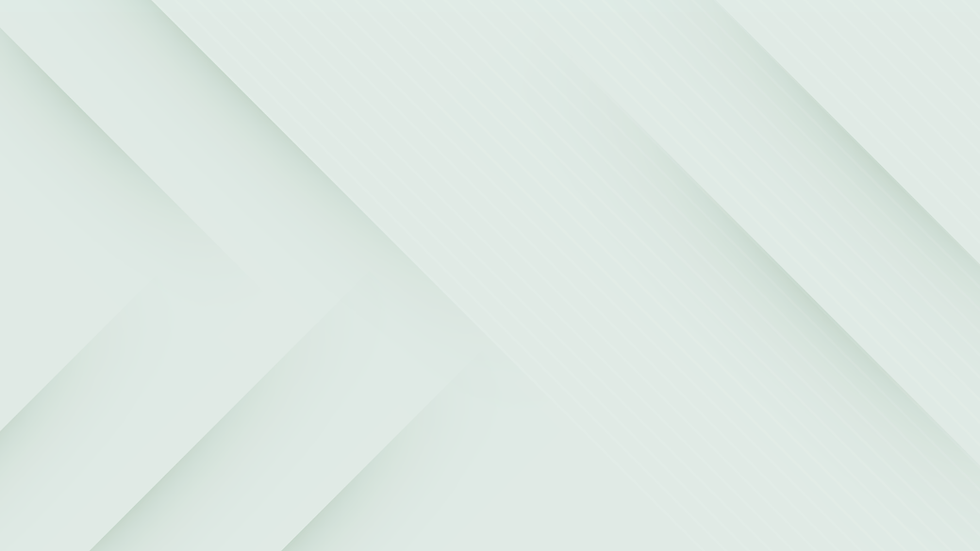
0 %
0 out of 5 lessons completed
FX Options Builder: Simplifying Currency Risk Management
Coming Soon...
Coming Soon...
Coming Soon...
Advanced Strategy Simulation
3 minutes reading
Introduction
Monte Carlo simulation is a very well-established approach to modeling situations with significant uncertainty; it can provide approximate solutions to problems that are otherwise intractable. In our context, MC methods are used to model the FX spot market in order to determine the relative efficacy of various hedging products and enable the hedger to identify the best one. As it turns out, there is a simplistic implementation that gives useful but incomplete results and more complex methods that reflect how the market works. Let’s examine each in turn.
The simplistic method
The most common implementation of Monte Carlo simulation in finance uses a distribution called Geometric Brownian Motion (GBM). It uses several parameters to control the paths generated: an initial value, a drift and a volatility in conjunction with a random number generator to generate a series of points that make up each path (a ‘random walk’). Finance borrowed this concept from fluid mechanics, where the diffusion equation (courtesy of Einstein, BTW) describes the motion of particles in a liquid. Unlike fluids, though, a direct BM is not appropriate for modeling FX. Instead, a Geometric Brownian is used so there will be no negative numbers (e.g. a ‘log normal” distribution.)
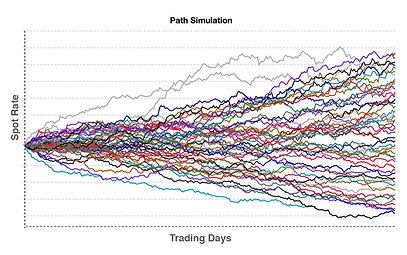

GBM can be very useful, but has a fatal flaw – it assumes that volatility is constant and that future prices are uncorrelated across time. Those assumptions are rather naïve (ever heard of meme stocks? Momentum trading?). Fig 2 shows a real-world distribution of USDJPY spot for one year- clearly not a log-normal distribution about a mean.
​
So GBM is convenient, but not realistic.
How can we do better?
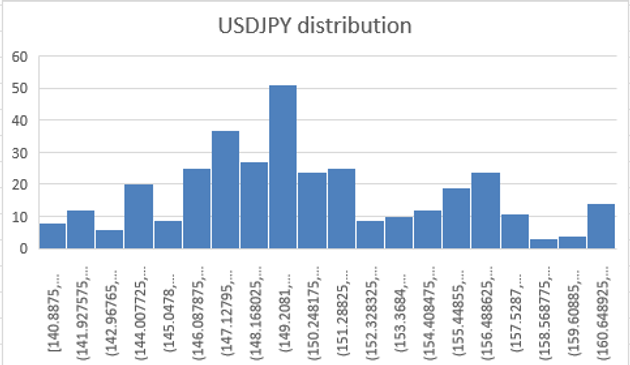
Stochastic volatility – welcome to the real world
One huge improvement to GBM is to toss the (very sketchy) assumption of constant volatility and allow it to vary during the course of each path. ‘Stochastic’ means that a variable is randomly determined, and can’t be predicted precisely. Instead, a probability distribution can be developed instead. What this means in our case is that volatility has a distribution – it will have a mean and volatility of its own – a vol of vol, so to speak.
​
The most common model for this is the Heston model. In addition to the vol of vol, it introduces two additional real-world characteristics:
​
-
A correlation between the asset price and the vol (i.e. as the prices rise, so does the volatility).
-
Volatility returns to a long-term mean over time.
​
To use this model, the user needs to specify the volatility’s mean and std deviation, the correlation between asset and volatility, and the mean reversion factor (how fast does vol revert to the long-term mean.)
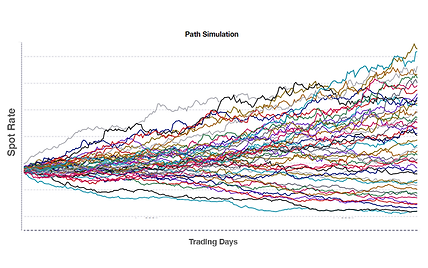
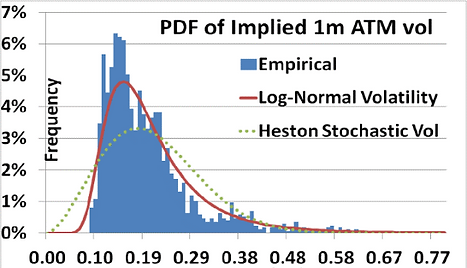
Jump diffusion – simulating catastrophe
There is a concept, popularized by Nassim Taleb, called a Black Swan. Black Swans are events that are unforeseen and carry a hugecarry huge impact. They can be positive: the rise of the internet, or the Arab Spring. Usually in finance they refer to negative events, such as 9/11, the Financial Crisis of 2008, or the COVID-19 outbreak. Typically couched in terms of the ‘hundred-year event’, they obviously happen much more frequently, and cause huge dislocations in markets. For example, GBP lost more than 30% of its value against USD in just a few weeks during the 2008 crash.
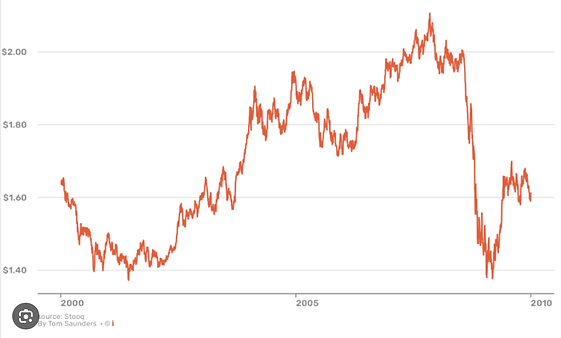
Merton developed a method of simulating these processes by adding a random term to the market modeling equation. We have implemented the Merton process to create a spot simulation with jump discontinuities, as can be seen in Fig 5. As always, with these more complicated models, additional parameters are needed to control the process. For jump diffusion, these include: mean of the jump size, std deviation of the jump size, and finally the intensity of the jump (number of jumps per annum).
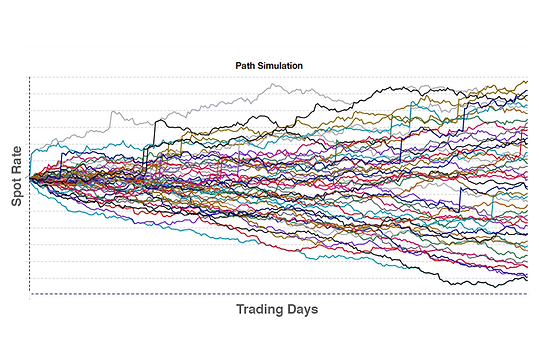
Conclusion
There are many ways to simulate a market in order to evaluate risk management strategies. There is a danger in using models which do not account for real-world effects, such as vol of vol or Black Swans. Many structured option products (e.g. Seagull) exhibit heightened tail risk when unexpected market behavior arises. If your strategy is sensitive to these effects, it may fail just when you need it the most. Testing your strategy against more accurate models will ensure no nasty surprises.
Hedge Smart, Risk Less
Members:
I
+201 312 6833
© 2024 Deaglo Inc. All Rights Reserved. Deaglo Inc. is a company registered in the United States (registered no. 88-2693379). 1078, Summit Ave. PMB 837, Jersey City, NJ 07307.
​
Deaglo, Inc. (“Deaglo”) is a registered Commodity Trading Advisor with the U.S. Commodity Futures Trading Commission (CFTC) and a Member of the National Futures Association (NFA). Deaglo’s CTA registration is effective according to an exemption that limits clients to those who are Qualified Eligible Persons as defined in CFTC Regulation 4.7.
Futures, options, FX, and swaps trading involve substantial risk and are not suitable for all clients. Therefore, clients should carefully consider their financial condition before deciding whether to invest and transact in these markets. PAST PERFORMANCE IS NOT INDICATIVE OF FUTURE RESULTS.
​
This material should only be considered current as of the date indicated on each page of data without regard to the date on which you may access the information. Deaglo maintains the right to delete or modify information without prior written notice.